Fritz™ scans & ranks all AI tools
We are developing an AI that analyzes all the tools, apps, and websites under our supervision, and ranks the absolute best and most ethical.
Check out our technical guides, reviews and tutorials to get started with machine learning.
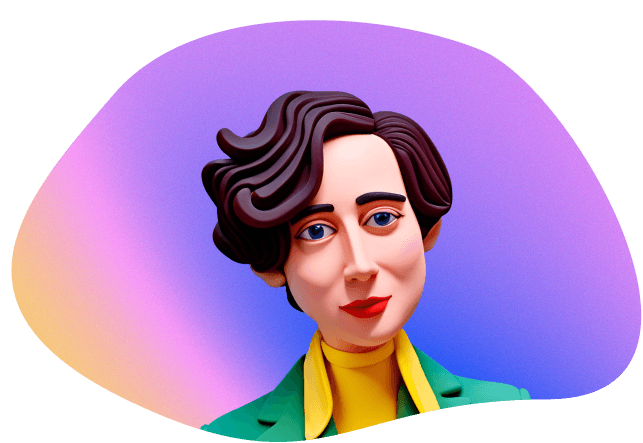
As featured in…
Who is Fritz?
Fritz is an LLM trained to help us aggregate and analyze the best AI tools, according to a set of criteria we decided to be crucial for this new generation of software.
AI Ethics
The main thing we care about! We only recommend apps with solid ethics.
Functionality & UX
Then we carefully review the functionality of each tool and the user experience.
Innovation
And finally, we consider the originality factor: does it have some oumph?
Most popular articles
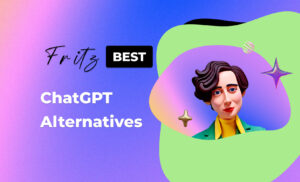
ChatGPT Alternatives
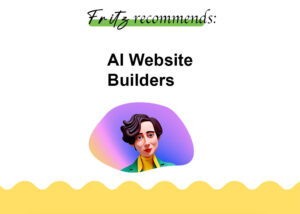
AI Website Builders
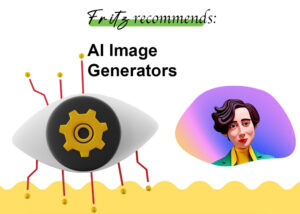
AI Image Generators
aiPDF Review
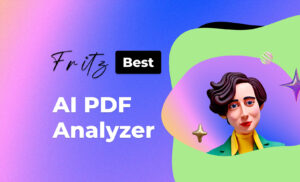
AI PDF Analyzer
Frequently Asked Questions
Fritz AI is basically your AI best buddy, guiding you through the maze of AI tools and apps out there. We review, rank, and break down the tech to help you get started or level up your AI game.
Our secret sauce? A mix of hands-on testing, community input, and keeping tabs on the latest in AI research. We look at how user-friendly, innovative, and ethically designed each tool is, then we check how people are using and reviewing them in real time.
Absolutely. We’ve crafted a bunch of beginner-friendly guides and tutorials to ease you into AI and machine learning, making sure you’ve got a solid starting point.
Definitely! If you’ve got a tool you love or an idea you’re excited about, let us know. We’re all about community collaboration.
Definitely! If you’ve got a tool you love or an idea you’re excited about, shoot us an email on hello at fritz.ai
Sure thing. Reach out via our contact page. We’re open to questions, collaboration ideas, or just a good old chat about AI.
Beyond our main gig of reviews and tutorials, we occasionally dive into consulting or collaborate on AI projects, helping businesses and enthusiasts alike navigate the AI space. Reach out via our contact page and we’ll do our best to help.
Ethics is a big deal for us. We promote the use of AI in ways that respect privacy, fairness, and accountability, making sure the tech is used for the greater good.